Getting Started¶
(c) 2021 Daniel Grose
Using python packages¶
To import a complete package into your python session or program you can use import. For example :
import time
This imports the time package and all of its functions, classes, data and global variables. For example, the time package has the function perf_counter. Try running the following python code to see what it does.
print(time.perf_counter())
118556.374832544
You can also import specific parts of a libray, for example, the random package has a random function
from random import random
print(random())
0.7907145070502625
from random import seed
seed(0)
print(random())
seed(0)
print(random())
0.8444218515250481
0.8444218515250481
Exercise 1¶
Generate a python list containing 100 random numbers
Solution 1.1¶
X = []
for i in range(100) :
X = X + [random()]
print(X)
[0.7579544029403025, 0.420571580830845, 0.25891675029296335, 0.5112747213686085, 0.4049341374504143, 0.7837985890347726, 0.30331272607892745, 0.4765969541523558, 0.5833820394550312, 0.9081128851953352, 0.5046868558173903, 0.28183784439970383, 0.7558042041572239, 0.6183689966753316, 0.25050634136244054, 0.9097462559682401, 0.9827854760376531, 0.8102172359965896, 0.9021659504395827, 0.3101475693193326, 0.7298317482601286, 0.8988382879679935, 0.6839839319154413, 0.47214271545271336, 0.1007012080683658, 0.4341718354537837, 0.6108869734438016, 0.9130110532378982, 0.9666063677707588, 0.47700977655271704, 0.8653099277716401, 0.2604923103919594, 0.8050278270130223, 0.5486993038355893, 0.014041700164018955, 0.7197046864039541, 0.39882354222426875, 0.824844977148233, 0.6681532012318508, 0.0011428193144282783, 0.49357786646532464, 0.8676027754927809, 0.24391087688713198, 0.32520436274739006, 0.8704712321086546, 0.19106709150239054, 0.5675107406206719, 0.23861592861522019, 0.9675402502901433, 0.80317946927987, 0.44796957143557037, 0.08044581855253541, 0.32005460467254576, 0.5079406425205739, 0.9328338242269067, 0.10905784593110368, 0.5512672460905512, 0.7065614098668896, 0.5474409113284238, 0.814466863291336, 0.540283606970324, 0.9638385459738009, 0.603185627961383, 0.5876170641754364, 0.4449890262755162, 0.5962868615831063, 0.38490114597266045, 0.5756510141648885, 0.290329502402758, 0.18939132855435614, 0.1867295282555551, 0.6127731798686067, 0.6566593889896288, 0.47653099200938076, 0.08982436119559367, 0.7576039219664368, 0.8767703708227748, 0.9233810159462806, 0.8424602231401824, 0.898173121357879, 0.9230824398201768, 0.5405999249480544, 0.3912960502346249, 0.7052833998544062, 0.27563412131212717, 0.8116287085078785, 0.8494859651863671, 0.8950389674266752, 0.5898011835311598, 0.9497648732321206, 0.5796950107456059, 0.4505631066311552, 0.660245378622389, 0.9962578393535727, 0.9169412179474561, 0.7933250841302242, 0.0823729881966474, 0.6127831050407122, 0.4864442019691668, 0.6301473404114728]
Solution 1.2¶
X = [random() for i in range(100)]
print(X)
[0.8450775756715152, 0.24303562206185625, 0.7314892207908478, 0.11713429320851798, 0.22046053686782852, 0.7945829717105759, 0.33253614921965546, 0.8159130965336595, 0.1006075202160962, 0.14635848891230385, 0.6976706401912388, 0.04523406786561235, 0.5738660367891669, 0.9100160146990397, 0.534197968260724, 0.6805891325622565, 0.026696794662205203, 0.6349999099114583, 0.6063384177542189, 0.5759529480315407, 0.3912094093228269, 0.3701399403351875, 0.9805166506472687, 0.036392037611485795, 0.021636509855024078, 0.9610312802396112, 0.18497194139743833, 0.12389516442443171, 0.21057650988664645, 0.8007465903541809, 0.9369691586445807, 0.022782575668658378, 0.42561883196681716, 0.10150021937416975, 0.259919889792832, 0.22082927131631735, 0.6469257198353225, 0.3502939673965323, 0.18031790152968785, 0.5036365052098872, 0.03937870708469238, 0.10092124118896661, 0.9882351487225011, 0.19935579046706298, 0.35855530131160185, 0.7315983062253606, 0.8383265651934163, 0.9184820619953314, 0.16942460609746768, 0.6726405635730526, 0.9665489030431832, 0.05805094382649867, 0.6762017842993783, 0.8454245937016164, 0.342312541078584, 0.25068733928511167, 0.596791393469411, 0.44231403369907896, 0.17481948445144113, 0.47162541509628797, 0.40990539565755457, 0.5691127395242802, 0.5086001300626332, 0.3114460010002068, 0.35715168259026286, 0.837661174368979, 0.25093266482213705, 0.560600218853524, 0.012436318829314397, 0.7415743774106636, 0.3359165544734606, 0.04569649356841665, 0.28088316421834825, 0.24013040782635398, 0.9531293398277989, 0.35222556151550743, 0.2878779148564, 0.35920119725374633, 0.9469058356578911, 0.6337478522492526, 0.6210768456186673, 0.7156193503014563, 0.38801723531250565, 0.4144179882772473, 0.650832862263345, 0.001524221856720187, 0.1923095412446758, 0.3344016906625016, 0.23941596018595857, 0.6373994011293003, 0.37864807032309444, 0.8754233917130172, 0.5681514209101919, 0.4144063966836443, 0.40226707511907955, 0.7018296239336754, 0.41822655329246605, 0.6621958889738174, 0.04677968595679827, 0.44535218971882984]
Some useful functions do not need to be imported, they come as standard. For example, the sort function
X.sort()
print(X)
[0.001524221856720187, 0.012436318829314397, 0.021636509855024078, 0.022782575668658378, 0.026696794662205203, 0.036392037611485795, 0.03937870708469238, 0.04523406786561235, 0.04569649356841665, 0.04677968595679827, 0.05805094382649867, 0.1006075202160962, 0.10092124118896661, 0.10150021937416975, 0.11713429320851798, 0.12389516442443171, 0.14635848891230385, 0.16942460609746768, 0.17481948445144113, 0.18031790152968785, 0.18497194139743833, 0.1923095412446758, 0.19935579046706298, 0.21057650988664645, 0.22046053686782852, 0.22082927131631735, 0.23941596018595857, 0.24013040782635398, 0.24303562206185625, 0.25068733928511167, 0.25093266482213705, 0.259919889792832, 0.28088316421834825, 0.2878779148564, 0.3114460010002068, 0.33253614921965546, 0.3344016906625016, 0.3359165544734606, 0.342312541078584, 0.3502939673965323, 0.35222556151550743, 0.35715168259026286, 0.35855530131160185, 0.35920119725374633, 0.3701399403351875, 0.37864807032309444, 0.38801723531250565, 0.3912094093228269, 0.40226707511907955, 0.40990539565755457, 0.4144063966836443, 0.4144179882772473, 0.41822655329246605, 0.42561883196681716, 0.44231403369907896, 0.44535218971882984, 0.47162541509628797, 0.5036365052098872, 0.5086001300626332, 0.534197968260724, 0.560600218853524, 0.5681514209101919, 0.5691127395242802, 0.5738660367891669, 0.5759529480315407, 0.596791393469411, 0.6063384177542189, 0.6210768456186673, 0.6337478522492526, 0.6349999099114583, 0.6373994011293003, 0.6469257198353225, 0.650832862263345, 0.6621958889738174, 0.6726405635730526, 0.6762017842993783, 0.6805891325622565, 0.6976706401912388, 0.7018296239336754, 0.7156193503014563, 0.7314892207908478, 0.7315983062253606, 0.7415743774106636, 0.7945829717105759, 0.8007465903541809, 0.8159130965336595, 0.837661174368979, 0.8383265651934163, 0.8450775756715152, 0.8454245937016164, 0.8754233917130172, 0.9100160146990397, 0.9184820619953314, 0.9369691586445807, 0.9469058356578911, 0.9531293398277989, 0.9610312802396112, 0.9665489030431832, 0.9805166506472687, 0.9882351487225011]
Exercise 2¶
Use the functions introduced above to find out how long it takes to sort a list of 100000 random numbers.
Solution 2.1¶
seed(0)
X = [random() for i in range(100000)]
start_time = time.perf_counter()
X.sort()
end_time = time.perf_counter()
print(end_time - start_time)
0.02980439599195961
Exercise 3¶
How does the time it takes to sort a list of random numbers change with the size of the list ? Write some code to find out.
Solution 3.1¶
t = []
for i in range(20) :
seed(0)
X = [random() for i in range(1000*i)]
start_time = time.perf_counter()
X.sort()
end_time = time.perf_counter()
t = t + [end_time - start_time]
print(t)
[2.6560010155662894e-06, 0.0003465899935690686, 0.00035856499744113535, 0.0005655249988194555, 0.0007856379961594939, 0.0009915859991451725, 0.0012224419915582985, 0.001474748001783155, 0.0017137030081357807, 0.0019906739908037707, 0.002194169006543234, 0.0024319570075022057, 0.0027498749986989424, 0.0029486809944501147, 0.0032629970082780346, 0.003521669001202099, 0.0037817130069015548, 0.004026811002404429, 0.004282334994059056, 0.004657345998566598]
Installing python packages with pip¶
Maybe it is not clear from just looking at the data what the relationship between the size of the list and the time taken to sort it is. There is a python package for plotting which could be of assistance with this. The package, matplotlib, is really quite powerful, but the package does not come as part of python. It has to be installed from PyPI (the Python Package Index) using pip. Normally, you could do this using a shell or command line using
python3 -m pip install matplotlib
You can do the equivalent of this in Jupyter Notebook by typing the same command with a ! added to the front of it.
!python3 -m pip install matplotlib
Requirement already satisfied: matplotlib in /home/grosedj/python-envs/further-python-env/env/lib/python3.9/site-packages (3.4.1)
Requirement already satisfied: cycler>=0.10 in /home/grosedj/python-envs/further-python-env/env/lib/python3.9/site-packages (from matplotlib) (0.10.0)
Requirement already satisfied: python-dateutil>=2.7 in /home/grosedj/python-envs/further-python-env/env/lib/python3.9/site-packages (from matplotlib) (2.8.1)
Requirement already satisfied: numpy>=1.16 in /home/grosedj/python-envs/further-python-env/env/lib/python3.9/site-packages (from matplotlib) (1.20.2)
Requirement already satisfied: pyparsing>=2.2.1 in /home/grosedj/python-envs/further-python-env/env/lib/python3.9/site-packages (from matplotlib) (2.4.7)
Requirement already satisfied: kiwisolver>=1.0.1 in /home/grosedj/python-envs/further-python-env/env/lib/python3.9/site-packages (from matplotlib) (1.3.1)
Requirement already satisfied: pillow>=6.2.0 in /home/grosedj/python-envs/further-python-env/env/lib/python3.9/site-packages (from matplotlib) (8.2.0)
Requirement already satisfied: six in /home/grosedj/python-envs/further-python-env/env/lib/python3.9/site-packages (from cycler>=0.10->matplotlib) (1.15.0)
WARNING: You are using pip version 20.2.3; however, version 21.0.1 is available.
You should consider upgrading via the '/home/grosedj/python-envs/further-python-env/env/bin/python3 -m pip install --upgrade pip' command.
The matplotlib package can now be imported. However, it is quite big, so it is a good idea to just import the functions that are needed. For creating a simple line plot the pyplot module can be used.
from matplotlib import pyplot as plt
Notice how this was imported. The above line of python can be interpreted as - from the matplotlib package import the module pyplot and rename it as plt. The methods associated matplotlib.pyplot can be used through plt as shown in the next example
plt.plot(t)
plt.show()
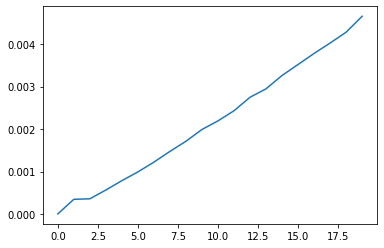
Exercise 4¶
Use matplotlib to explore the relationship between the length of the list and the time taken to sort it.
Maybe a random sequence of values is not the best way to do this. What other structure for the data to be sorted might be useful to consider ?
What do the results look like when you try these as input to the sort function ?
Solution 4.1¶
t = []
for i in range(20) :
seed(0)
X = list(range(10000*i))
# X.reverse()
start_time = time.perf_counter()
X.sort()
end_time = time.perf_counter()
t = t + [end_time - start_time]
plt.plot(t)
plt.show()
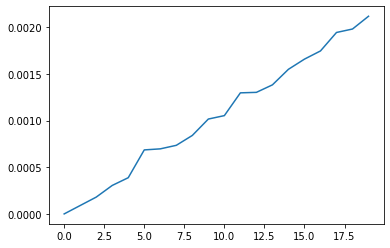
Exercise 5¶
Does your plot have any spikes in it ? If so, why is this the case ?
Exercise 6¶
The chapter Using Matplotlib explains some of the features of matplotlib in more detail. Have a quick look through it and see if you can find any ways of improving the plots you have been using.
Geting help¶
There are several ways of getting help in an interactive python session. For example, to get help on a package you can use the following. Note, the argument passed to the help function is a string.
help("random")
Help on module random:
NAME
random - Random variable generators.
MODULE REFERENCE
https://docs.python.org/3.9/library/random
The following documentation is automatically generated from the Python
source files. It may be incomplete, incorrect or include features that
are considered implementation detail and may vary between Python
implementations. When in doubt, consult the module reference at the
location listed above.
DESCRIPTION
bytes
-----
uniform bytes (values between 0 and 255)
integers
--------
uniform within range
sequences
---------
pick random element
pick random sample
pick weighted random sample
generate random permutation
distributions on the real line:
------------------------------
uniform
triangular
normal (Gaussian)
lognormal
negative exponential
gamma
beta
pareto
Weibull
distributions on the circle (angles 0 to 2pi)
---------------------------------------------
circular uniform
von Mises
General notes on the underlying Mersenne Twister core generator:
* The period is 2**19937-1.
* It is one of the most extensively tested generators in existence.
* The random() method is implemented in C, executes in a single Python step,
and is, therefore, threadsafe.
CLASSES
_random.Random(builtins.object)
Random
SystemRandom
class Random(_random.Random)
| Random(x=None)
|
| Random number generator base class used by bound module functions.
|
| Used to instantiate instances of Random to get generators that don't
| share state.
|
| Class Random can also be subclassed if you want to use a different basic
| generator of your own devising: in that case, override the following
| methods: random(), seed(), getstate(), and setstate().
| Optionally, implement a getrandbits() method so that randrange()
| can cover arbitrarily large ranges.
|
| Method resolution order:
| Random
| _random.Random
| builtins.object
|
| Methods defined here:
|
| __getstate__(self)
| # Issue 17489: Since __reduce__ was defined to fix #759889 this is no
| # longer called; we leave it here because it has been here since random was
| # rewritten back in 2001 and why risk breaking something.
|
| __init__(self, x=None)
| Initialize an instance.
|
| Optional argument x controls seeding, as for Random.seed().
|
| __reduce__(self)
| Helper for pickle.
|
| __setstate__(self, state)
|
| betavariate(self, alpha, beta)
| Beta distribution.
|
| Conditions on the parameters are alpha > 0 and beta > 0.
| Returned values range between 0 and 1.
|
| choice(self, seq)
| Choose a random element from a non-empty sequence.
|
| choices(self, population, weights=None, *, cum_weights=None, k=1)
| Return a k sized list of population elements chosen with replacement.
|
| If the relative weights or cumulative weights are not specified,
| the selections are made with equal probability.
|
| expovariate(self, lambd)
| Exponential distribution.
|
| lambd is 1.0 divided by the desired mean. It should be
| nonzero. (The parameter would be called "lambda", but that is
| a reserved word in Python.) Returned values range from 0 to
| positive infinity if lambd is positive, and from negative
| infinity to 0 if lambd is negative.
|
| gammavariate(self, alpha, beta)
| Gamma distribution. Not the gamma function!
|
| Conditions on the parameters are alpha > 0 and beta > 0.
|
| The probability distribution function is:
|
| x ** (alpha - 1) * math.exp(-x / beta)
| pdf(x) = --------------------------------------
| math.gamma(alpha) * beta ** alpha
|
| gauss(self, mu, sigma)
| Gaussian distribution.
|
| mu is the mean, and sigma is the standard deviation. This is
| slightly faster than the normalvariate() function.
|
| Not thread-safe without a lock around calls.
|
| getstate(self)
| Return internal state; can be passed to setstate() later.
|
| lognormvariate(self, mu, sigma)
| Log normal distribution.
|
| If you take the natural logarithm of this distribution, you'll get a
| normal distribution with mean mu and standard deviation sigma.
| mu can have any value, and sigma must be greater than zero.
|
| normalvariate(self, mu, sigma)
| Normal distribution.
|
| mu is the mean, and sigma is the standard deviation.
|
| paretovariate(self, alpha)
| Pareto distribution. alpha is the shape parameter.
|
| randbytes(self, n)
| Generate n random bytes.
|
| randint(self, a, b)
| Return random integer in range [a, b], including both end points.
|
| randrange(self, start, stop=None, step=1)
| Choose a random item from range(start, stop[, step]).
|
| This fixes the problem with randint() which includes the
| endpoint; in Python this is usually not what you want.
|
| sample(self, population, k, *, counts=None)
| Chooses k unique random elements from a population sequence or set.
|
| Returns a new list containing elements from the population while
| leaving the original population unchanged. The resulting list is
| in selection order so that all sub-slices will also be valid random
| samples. This allows raffle winners (the sample) to be partitioned
| into grand prize and second place winners (the subslices).
|
| Members of the population need not be hashable or unique. If the
| population contains repeats, then each occurrence is a possible
| selection in the sample.
|
| Repeated elements can be specified one at a time or with the optional
| counts parameter. For example:
|
| sample(['red', 'blue'], counts=[4, 2], k=5)
|
| is equivalent to:
|
| sample(['red', 'red', 'red', 'red', 'blue', 'blue'], k=5)
|
| To choose a sample from a range of integers, use range() for the
| population argument. This is especially fast and space efficient
| for sampling from a large population:
|
| sample(range(10000000), 60)
|
| seed(self, a=None, version=2)
| Initialize internal state from a seed.
|
| The only supported seed types are None, int, float,
| str, bytes, and bytearray.
|
| None or no argument seeds from current time or from an operating
| system specific randomness source if available.
|
| If *a* is an int, all bits are used.
|
| For version 2 (the default), all of the bits are used if *a* is a str,
| bytes, or bytearray. For version 1 (provided for reproducing random
| sequences from older versions of Python), the algorithm for str and
| bytes generates a narrower range of seeds.
|
| setstate(self, state)
| Restore internal state from object returned by getstate().
|
| shuffle(self, x, random=None)
| Shuffle list x in place, and return None.
|
| Optional argument random is a 0-argument function returning a
| random float in [0.0, 1.0); if it is the default None, the
| standard random.random will be used.
|
| triangular(self, low=0.0, high=1.0, mode=None)
| Triangular distribution.
|
| Continuous distribution bounded by given lower and upper limits,
| and having a given mode value in-between.
|
| http://en.wikipedia.org/wiki/Triangular_distribution
|
| uniform(self, a, b)
| Get a random number in the range [a, b) or [a, b] depending on rounding.
|
| vonmisesvariate(self, mu, kappa)
| Circular data distribution.
|
| mu is the mean angle, expressed in radians between 0 and 2*pi, and
| kappa is the concentration parameter, which must be greater than or
| equal to zero. If kappa is equal to zero, this distribution reduces
| to a uniform random angle over the range 0 to 2*pi.
|
| weibullvariate(self, alpha, beta)
| Weibull distribution.
|
| alpha is the scale parameter and beta is the shape parameter.
|
| ----------------------------------------------------------------------
| Class methods defined here:
|
| __init_subclass__(**kwargs) from builtins.type
| Control how subclasses generate random integers.
|
| The algorithm a subclass can use depends on the random() and/or
| getrandbits() implementation available to it and determines
| whether it can generate random integers from arbitrarily large
| ranges.
|
| ----------------------------------------------------------------------
| Data descriptors defined here:
|
| __dict__
| dictionary for instance variables (if defined)
|
| __weakref__
| list of weak references to the object (if defined)
|
| ----------------------------------------------------------------------
| Data and other attributes defined here:
|
| VERSION = 3
|
| ----------------------------------------------------------------------
| Methods inherited from _random.Random:
|
| getrandbits(self, k, /)
| getrandbits(k) -> x. Generates an int with k random bits.
|
| random(self, /)
| random() -> x in the interval [0, 1).
|
| ----------------------------------------------------------------------
| Static methods inherited from _random.Random:
|
| __new__(*args, **kwargs) from builtins.type
| Create and return a new object. See help(type) for accurate signature.
class SystemRandom(Random)
| SystemRandom(x=None)
|
| Alternate random number generator using sources provided
| by the operating system (such as /dev/urandom on Unix or
| CryptGenRandom on Windows).
|
| Not available on all systems (see os.urandom() for details).
|
| Method resolution order:
| SystemRandom
| Random
| _random.Random
| builtins.object
|
| Methods defined here:
|
| getrandbits(self, k)
| getrandbits(k) -> x. Generates an int with k random bits.
|
| getstate = _notimplemented(self, *args, **kwds)
|
| randbytes(self, n)
| Generate n random bytes.
|
| random(self)
| Get the next random number in the range [0.0, 1.0).
|
| seed(self, *args, **kwds)
| Stub method. Not used for a system random number generator.
|
| setstate = _notimplemented(self, *args, **kwds)
|
| ----------------------------------------------------------------------
| Methods inherited from Random:
|
| __getstate__(self)
| # Issue 17489: Since __reduce__ was defined to fix #759889 this is no
| # longer called; we leave it here because it has been here since random was
| # rewritten back in 2001 and why risk breaking something.
|
| __init__(self, x=None)
| Initialize an instance.
|
| Optional argument x controls seeding, as for Random.seed().
|
| __reduce__(self)
| Helper for pickle.
|
| __setstate__(self, state)
|
| betavariate(self, alpha, beta)
| Beta distribution.
|
| Conditions on the parameters are alpha > 0 and beta > 0.
| Returned values range between 0 and 1.
|
| choice(self, seq)
| Choose a random element from a non-empty sequence.
|
| choices(self, population, weights=None, *, cum_weights=None, k=1)
| Return a k sized list of population elements chosen with replacement.
|
| If the relative weights or cumulative weights are not specified,
| the selections are made with equal probability.
|
| expovariate(self, lambd)
| Exponential distribution.
|
| lambd is 1.0 divided by the desired mean. It should be
| nonzero. (The parameter would be called "lambda", but that is
| a reserved word in Python.) Returned values range from 0 to
| positive infinity if lambd is positive, and from negative
| infinity to 0 if lambd is negative.
|
| gammavariate(self, alpha, beta)
| Gamma distribution. Not the gamma function!
|
| Conditions on the parameters are alpha > 0 and beta > 0.
|
| The probability distribution function is:
|
| x ** (alpha - 1) * math.exp(-x / beta)
| pdf(x) = --------------------------------------
| math.gamma(alpha) * beta ** alpha
|
| gauss(self, mu, sigma)
| Gaussian distribution.
|
| mu is the mean, and sigma is the standard deviation. This is
| slightly faster than the normalvariate() function.
|
| Not thread-safe without a lock around calls.
|
| lognormvariate(self, mu, sigma)
| Log normal distribution.
|
| If you take the natural logarithm of this distribution, you'll get a
| normal distribution with mean mu and standard deviation sigma.
| mu can have any value, and sigma must be greater than zero.
|
| normalvariate(self, mu, sigma)
| Normal distribution.
|
| mu is the mean, and sigma is the standard deviation.
|
| paretovariate(self, alpha)
| Pareto distribution. alpha is the shape parameter.
|
| randint(self, a, b)
| Return random integer in range [a, b], including both end points.
|
| randrange(self, start, stop=None, step=1)
| Choose a random item from range(start, stop[, step]).
|
| This fixes the problem with randint() which includes the
| endpoint; in Python this is usually not what you want.
|
| sample(self, population, k, *, counts=None)
| Chooses k unique random elements from a population sequence or set.
|
| Returns a new list containing elements from the population while
| leaving the original population unchanged. The resulting list is
| in selection order so that all sub-slices will also be valid random
| samples. This allows raffle winners (the sample) to be partitioned
| into grand prize and second place winners (the subslices).
|
| Members of the population need not be hashable or unique. If the
| population contains repeats, then each occurrence is a possible
| selection in the sample.
|
| Repeated elements can be specified one at a time or with the optional
| counts parameter. For example:
|
| sample(['red', 'blue'], counts=[4, 2], k=5)
|
| is equivalent to:
|
| sample(['red', 'red', 'red', 'red', 'blue', 'blue'], k=5)
|
| To choose a sample from a range of integers, use range() for the
| population argument. This is especially fast and space efficient
| for sampling from a large population:
|
| sample(range(10000000), 60)
|
| shuffle(self, x, random=None)
| Shuffle list x in place, and return None.
|
| Optional argument random is a 0-argument function returning a
| random float in [0.0, 1.0); if it is the default None, the
| standard random.random will be used.
|
| triangular(self, low=0.0, high=1.0, mode=None)
| Triangular distribution.
|
| Continuous distribution bounded by given lower and upper limits,
| and having a given mode value in-between.
|
| http://en.wikipedia.org/wiki/Triangular_distribution
|
| uniform(self, a, b)
| Get a random number in the range [a, b) or [a, b] depending on rounding.
|
| vonmisesvariate(self, mu, kappa)
| Circular data distribution.
|
| mu is the mean angle, expressed in radians between 0 and 2*pi, and
| kappa is the concentration parameter, which must be greater than or
| equal to zero. If kappa is equal to zero, this distribution reduces
| to a uniform random angle over the range 0 to 2*pi.
|
| weibullvariate(self, alpha, beta)
| Weibull distribution.
|
| alpha is the scale parameter and beta is the shape parameter.
|
| ----------------------------------------------------------------------
| Class methods inherited from Random:
|
| __init_subclass__(**kwargs) from builtins.type
| Control how subclasses generate random integers.
|
| The algorithm a subclass can use depends on the random() and/or
| getrandbits() implementation available to it and determines
| whether it can generate random integers from arbitrarily large
| ranges.
|
| ----------------------------------------------------------------------
| Data descriptors inherited from Random:
|
| __dict__
| dictionary for instance variables (if defined)
|
| __weakref__
| list of weak references to the object (if defined)
|
| ----------------------------------------------------------------------
| Data and other attributes inherited from Random:
|
| VERSION = 3
|
| ----------------------------------------------------------------------
| Static methods inherited from _random.Random:
|
| __new__(*args, **kwargs) from builtins.type
| Create and return a new object. See help(type) for accurate signature.
FUNCTIONS
betavariate(alpha, beta) method of Random instance
Beta distribution.
Conditions on the parameters are alpha > 0 and beta > 0.
Returned values range between 0 and 1.
choice(seq) method of Random instance
Choose a random element from a non-empty sequence.
choices(population, weights=None, *, cum_weights=None, k=1) method of Random instance
Return a k sized list of population elements chosen with replacement.
If the relative weights or cumulative weights are not specified,
the selections are made with equal probability.
expovariate(lambd) method of Random instance
Exponential distribution.
lambd is 1.0 divided by the desired mean. It should be
nonzero. (The parameter would be called "lambda", but that is
a reserved word in Python.) Returned values range from 0 to
positive infinity if lambd is positive, and from negative
infinity to 0 if lambd is negative.
gammavariate(alpha, beta) method of Random instance
Gamma distribution. Not the gamma function!
Conditions on the parameters are alpha > 0 and beta > 0.
The probability distribution function is:
x ** (alpha - 1) * math.exp(-x / beta)
pdf(x) = --------------------------------------
math.gamma(alpha) * beta ** alpha
gauss(mu, sigma) method of Random instance
Gaussian distribution.
mu is the mean, and sigma is the standard deviation. This is
slightly faster than the normalvariate() function.
Not thread-safe without a lock around calls.
getrandbits(k, /) method of Random instance
getrandbits(k) -> x. Generates an int with k random bits.
getstate() method of Random instance
Return internal state; can be passed to setstate() later.
lognormvariate(mu, sigma) method of Random instance
Log normal distribution.
If you take the natural logarithm of this distribution, you'll get a
normal distribution with mean mu and standard deviation sigma.
mu can have any value, and sigma must be greater than zero.
normalvariate(mu, sigma) method of Random instance
Normal distribution.
mu is the mean, and sigma is the standard deviation.
paretovariate(alpha) method of Random instance
Pareto distribution. alpha is the shape parameter.
randint(a, b) method of Random instance
Return random integer in range [a, b], including both end points.
random() method of Random instance
random() -> x in the interval [0, 1).
randrange(start, stop=None, step=1) method of Random instance
Choose a random item from range(start, stop[, step]).
This fixes the problem with randint() which includes the
endpoint; in Python this is usually not what you want.
sample(population, k, *, counts=None) method of Random instance
Chooses k unique random elements from a population sequence or set.
Returns a new list containing elements from the population while
leaving the original population unchanged. The resulting list is
in selection order so that all sub-slices will also be valid random
samples. This allows raffle winners (the sample) to be partitioned
into grand prize and second place winners (the subslices).
Members of the population need not be hashable or unique. If the
population contains repeats, then each occurrence is a possible
selection in the sample.
Repeated elements can be specified one at a time or with the optional
counts parameter. For example:
sample(['red', 'blue'], counts=[4, 2], k=5)
is equivalent to:
sample(['red', 'red', 'red', 'red', 'blue', 'blue'], k=5)
To choose a sample from a range of integers, use range() for the
population argument. This is especially fast and space efficient
for sampling from a large population:
sample(range(10000000), 60)
seed(a=None, version=2) method of Random instance
Initialize internal state from a seed.
The only supported seed types are None, int, float,
str, bytes, and bytearray.
None or no argument seeds from current time or from an operating
system specific randomness source if available.
If *a* is an int, all bits are used.
For version 2 (the default), all of the bits are used if *a* is a str,
bytes, or bytearray. For version 1 (provided for reproducing random
sequences from older versions of Python), the algorithm for str and
bytes generates a narrower range of seeds.
setstate(state) method of Random instance
Restore internal state from object returned by getstate().
shuffle(x, random=None) method of Random instance
Shuffle list x in place, and return None.
Optional argument random is a 0-argument function returning a
random float in [0.0, 1.0); if it is the default None, the
standard random.random will be used.
triangular(low=0.0, high=1.0, mode=None) method of Random instance
Triangular distribution.
Continuous distribution bounded by given lower and upper limits,
and having a given mode value in-between.
http://en.wikipedia.org/wiki/Triangular_distribution
uniform(a, b) method of Random instance
Get a random number in the range [a, b) or [a, b] depending on rounding.
vonmisesvariate(mu, kappa) method of Random instance
Circular data distribution.
mu is the mean angle, expressed in radians between 0 and 2*pi, and
kappa is the concentration parameter, which must be greater than or
equal to zero. If kappa is equal to zero, this distribution reduces
to a uniform random angle over the range 0 to 2*pi.
weibullvariate(alpha, beta) method of Random instance
Weibull distribution.
alpha is the scale parameter and beta is the shape parameter.
DATA
__all__ = ['Random', 'SystemRandom', 'betavariate', 'choice', 'choices...
FILE
/home/grosedj/.pyenv/versions/3.9.0/lib/python3.9/random.py
You can also use help to find out about a particular function. For example.
help("random.seed")
Help on method seed in random:
random.seed = seed(a=None, version=2) method of random.Random instance
Initialize internal state from a seed.
The only supported seed types are None, int, float,
str, bytes, and bytearray.
None or no argument seeds from current time or from an operating
system specific randomness source if available.
If *a* is an int, all bits are used.
For version 2 (the default), all of the bits are used if *a* is a str,
bytes, or bytearray. For version 1 (provided for reproducing random
sequences from older versions of Python), the algorithm for str and
bytes generates a narrower range of seeds.
Exercise 7.¶
Use help to get help on help.
Solution 7.1¶
help("help")
Help on _Helper in module _sitebuiltins object:
help = class _Helper(builtins.object)
| Define the builtin 'help'.
|
| This is a wrapper around pydoc.help that provides a helpful message
| when 'help' is typed at the Python interactive prompt.
|
| Calling help() at the Python prompt starts an interactive help session.
| Calling help(thing) prints help for the python object 'thing'.
|
| Methods defined here:
|
| __call__(self, *args, **kwds)
| Call self as a function.
|
| __repr__(self)
| Return repr(self).
|
| ----------------------------------------------------------------------
| Data descriptors defined here:
|
| __dict__
| dictionary for instance variables (if defined)
|
| __weakref__
| list of weak references to the object (if defined)
Exercise 8¶
Use your solution to exercise 7 to start an interactive help session.
Solution 8.1¶
help()
Welcome to Python 3.9's help utility!
If this is your first time using Python, you should definitely check out
the tutorial on the Internet at https://docs.python.org/3.9/tutorial/.
Enter the name of any module, keyword, or topic to get help on writing
Python programs and using Python modules. To quit this help utility and
return to the interpreter, just type "quit".
To get a list of available modules, keywords, symbols, or topics, type
"modules", "keywords", "symbols", or "topics". Each module also comes
with a one-line summary of what it does; to list the modules whose name
or summary contain a given string such as "spam", type "modules spam".
---------------------------------------------------------------------------
StdinNotImplementedError Traceback (most recent call last)
<ipython-input-17-9778277b2191> in <module>
----> 1 help()
~/.pyenv/versions/3.9.0/lib/python3.9/_sitebuiltins.py in __call__(self, *args, **kwds)
101 def __call__(self, *args, **kwds):
102 import pydoc
--> 103 return pydoc.help(*args, **kwds)
~/.pyenv/versions/3.9.0/lib/python3.9/pydoc.py in __call__(self, request)
2002 else:
2003 self.intro()
-> 2004 self.interact()
2005 self.output.write('''
2006 You are now leaving help and returning to the Python interpreter.
~/.pyenv/versions/3.9.0/lib/python3.9/pydoc.py in interact(self)
2014 while True:
2015 try:
-> 2016 request = self.getline('help> ')
2017 if not request: break
2018 except (KeyboardInterrupt, EOFError):
~/.pyenv/versions/3.9.0/lib/python3.9/pydoc.py in getline(self, prompt)
2034 """Read one line, using input() when appropriate."""
2035 if self.input is sys.stdin:
-> 2036 return input(prompt)
2037 else:
2038 self.output.write(prompt)
~/python-envs/further-python-env/env/lib/python3.9/site-packages/ipykernel/kernelbase.py in raw_input(self, prompt)
843 """
844 if not self._allow_stdin:
--> 845 raise StdinNotImplementedError(
846 "raw_input was called, but this frontend does not support input requests."
847 )
StdinNotImplementedError: raw_input was called, but this frontend does not support input requests.
Exercise 9¶
How do you stop an interactive help session ?
help("bisect")
Help on module bisect:
NAME
bisect - Bisection algorithms.
MODULE REFERENCE
https://docs.python.org/3.9/library/bisect
The following documentation is automatically generated from the Python
source files. It may be incomplete, incorrect or include features that
are considered implementation detail and may vary between Python
implementations. When in doubt, consult the module reference at the
location listed above.
FUNCTIONS
bisect = bisect_right(a, x, lo=0, hi=None)
Return the index where to insert item x in list a, assuming a is sorted.
The return value i is such that all e in a[:i] have e <= x, and all e in
a[i:] have e > x. So if x already appears in the list, i points just
beyond the rightmost x already there
Optional args lo (default 0) and hi (default len(a)) bound the
slice of a to be searched.
bisect_left(a, x, lo=0, hi=None)
Return the index where to insert item x in list a, assuming a is sorted.
The return value i is such that all e in a[:i] have e < x, and all e in
a[i:] have e >= x. So if x already appears in the list, i points just
before the leftmost x already there.
Optional args lo (default 0) and hi (default len(a)) bound the
slice of a to be searched.
bisect_right(a, x, lo=0, hi=None)
Return the index where to insert item x in list a, assuming a is sorted.
The return value i is such that all e in a[:i] have e <= x, and all e in
a[i:] have e > x. So if x already appears in the list, i points just
beyond the rightmost x already there
Optional args lo (default 0) and hi (default len(a)) bound the
slice of a to be searched.
insort = insort_right(a, x, lo=0, hi=None)
Insert item x in list a, and keep it sorted assuming a is sorted.
If x is already in a, insert it to the right of the rightmost x.
Optional args lo (default 0) and hi (default len(a)) bound the
slice of a to be searched.
insort_left(a, x, lo=0, hi=None)
Insert item x in list a, and keep it sorted assuming a is sorted.
If x is already in a, insert it to the left of the leftmost x.
Optional args lo (default 0) and hi (default len(a)) bound the
slice of a to be searched.
insort_right(a, x, lo=0, hi=None)
Insert item x in list a, and keep it sorted assuming a is sorted.
If x is already in a, insert it to the right of the rightmost x.
Optional args lo (default 0) and hi (default len(a)) bound the
slice of a to be searched.
FILE
/home/grosedj/.pyenv/versions/3.9.0/lib/python3.9/bisect.py
Challenge 1¶
i) Use help to find out about the bisect module.
ii) Create a list of 100 random numbers, put them in ascending order, and then insert 0.5 whilst keeping the list in order.
iii) Repeat ii) but for different lengths of random sequences. Use your results to determine how the time taken to insert an element in a list changes with the length of the list.
Challenge 2¶
i) In an sorted list of n random numbers find the position to insert the value 0.5
ii) Repeat i) k times to explore how the insertion position for the value 0.5 varies.
iii) How does the way in which the insertion position for 0.5 varies vary with the length n of the sequence.
iv) Use matplotlib to visualise the results you obtain in parts ii) and iii).
Challenge 3¶
i) Install the python library numpy.
ii) Import the library as np.
iii) Find out what random number distributions are available in the np.random module.
iv) Generate some random numbers from a poisson distribution using the numpy.random module.
v) Make a histogram of the sample you generated in iv).
Assessment Question¶
This task will form part of your assessment for this module of STOR-601.
a) Write a python function that accepts two sorted lists of n numbers as arguments and returns the value of the median of the combined data from both lists. [5]
b) Determine how the time taken for your function to calculate the median varies with n. Demonstrate this visually using matplotlib. [5]
c) What is the complexity of the algorithm you used in part a) ? [2]
d) Is it possible to write a function for part a) that has better than O(n) worst case complexity ? Research this problem, and if it is possible, write a python function that realises better than O(n) worst case complexity. If you think that it is not possible, then write a short explanation as to why this is so. [5]
Note - if your answer to part a) is better then O(n) worst case, then this constitutes an answer to part d) as well.